- by THS:UKI Manager
- 24 June 2022
- Corporate Member News
These are exciting times for the floating wind industry. With testing and validation under way and turbine production approaching ramp-up stage, now is the ideal moment for floating wind developers to build their data strategy.
Below are our six top tips for developing a floating wind data strategy that can ensure projects remain on course throughout the development journey.
1. Apply the laws of physics
Floating wind technology is now moving from numerical models to physical proof of concept in challenging marine environments. Pilot prototypes gather many different types of data for analysis. When analysing the interrelationship between these datasets, taking a physics-based approach is a must, because correlation is not always a causation.
Without a solid understanding of physics, developers risk extrapolating the data erroneously and arriving at conclusions that are fundamentally flawed. We always recommend using domain knowledge in the interpretation of results.
2. Define the data strategy at the outset
The volume of data gathered on a floating wind project is colossal and can very quickly become unmanageable, so it’s wise to prioritise the creation of a robust data strategy at the outset. Clarify the data lineage: long before acquisition begins, establish the rules for data governance, acquisition, access, iterations, and storage. And communicate these rules clearly.
3. Acquire data cost-effectively
Don’t over-gather data – having a greater volume of data doesn’t necessarily mean that it’ll be possible to extract more value from it. Be frugal instead. For example, decisions on data frequency and data resolution will have an impact on cost.
Adding analytics as close as possible to where the data source is in the data acquisition strategy is another way of being frugal. Examples range from trivial sampling models to reduce the data bandwidth, size and latency, to more complex event detections, snapshot policies or numerical models derived from observed data.
It’s often worthwhile to look beyond sensor-based data acquisition. Building a static dataset by digitising legacy data may provide a far more cost-effective solution. For example, developers may decide to develop a smart policy that optimises the use of historic and real-time data across the four key areas: wind, water, structure and seabed.
4. Orchestrate the data
Data is acquired in chunks. Developers need to be able to analyse each chunk quickly, to respond to identified changes. Rather than waiting weeks for data to be converted into a workable format for analysis, we recommend creating a framework that orchestrates the data from the moment it’s acquired. Fugro is building a framework that empowers clients to include their strategy for data orchestration, so that acquired data is immediately available for analysis.
5. Empower end users
Rather than building a rigid digital platform that standardises every aspect of floating wind across the organisation, many developers are benefiting from ‘thinking local’ and embedding some flexibility into their system.
Examples include empowering end users to build no-code applications (for example, to create customised dashboards) without having to submit requests to their development stream or software supplier enabling them to use drag-and-drop interfaces, so they can explore and distil insights from the data for themselves.
6. Leverage knowledge-transfer models
Data variance can cause difficulties with automated routines, like machine learning and industrial algorithms that are used for quality control. For example, different makes or types of sensors may each represent a certain feature in their own way. There may be geographic variations too, like earthquakes in California or hurricanes in Japan.
Simply merging different datasets is not an option, so we recommend working out how they can complement each other instead. Leverage knowledge-transfer models to manage data variance effectively. For example, if you look at wind height versus wave height versus a certain pattern on the seafloor, it’s possible to create a representation learning model and apply it to another geographic location. Incorporating variance and knowledge transfer in the strategy will produce richer, more meaningful data.
In conclusion
Demonstrator projects are now being used to validate the floating wind design concepts. The resultant data will be hugely valuable in standardising and derisking the many thousands of floating wind turbines that will generate renewable energy around the world in the years to come.
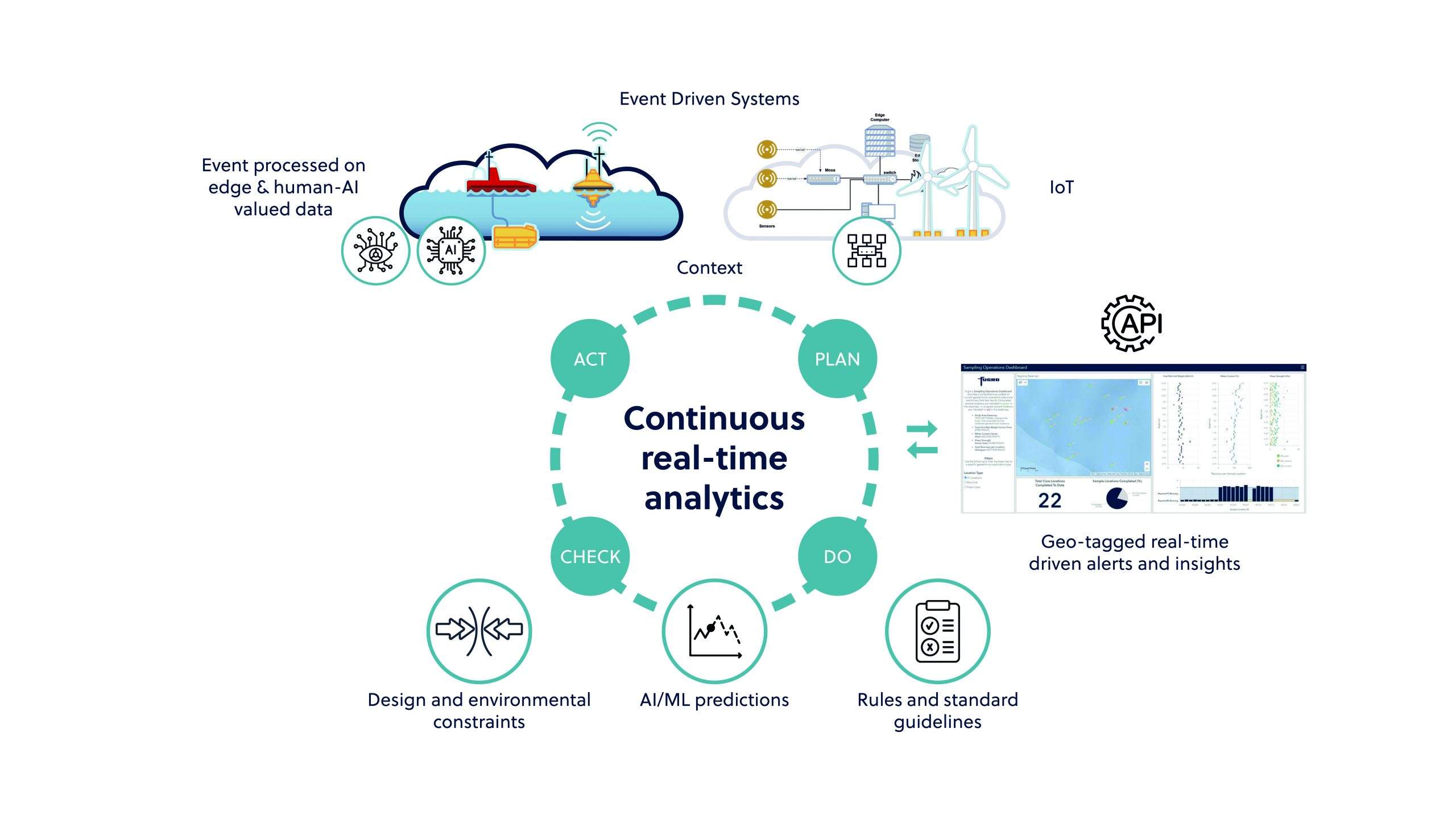
Fugro’s approach to data analytics
DID YOU KNOW?
The first offshore wind projects were designed using only a few megabytes of Geo-data, whereas modern projects use advanced and detailed datasets of 30 terabytes or more